In the ever-evolving banking industry landscape, staying ahead of the curve is essential for success. One area that has garnered increasing attention is provisioning models, which play a crucial role in managing risk and ensuring financial stability. But with an array of options to choose from, navigating the world of provisioning models can be overwhelming. Fear not, as this comprehensive guide is here to shed light on the subject, providing you with the knowledge and insights necessary to make informed decisions.
From the traditional incurred loss model to the more forward-looking expected credit loss model, we will explore the pros and cons of each approach, demystify complex terminology, and offer practical tips for implementation. Whether you are a banking professional looking to enhance your understanding or an executive seeking to optimise your institution's risk management strategies, this guide is your roadmap to success in the world of provisioning models.
Why provisioning models are important in banking
Provisioning models are crucial for banks as they allow for accurately measuring and managing credit risk. Banks face the challenge of balancing the need to provide credit to customers while ensuring their financial position stability. Provisioning models help in achieving this delicate balance by estimating the potential losses arising from credit risk and setting aside provisions to cover those losses.
These models are essential for several reasons. Firstly, they facilitate compliance with regulatory requirements. Regulators often require banks to maintain certain provisions to ensure the institution's financial stability and protect depositors' funds. Secondly, provisioning models enable banks to assess the creditworthiness of their borrowers and make informed lending decisions. By incorporating expected losses into their risk assessments, banks can better evaluate the potential risks associated with lending to different customers. Lastly, provisioning models provide valuable insights into the overall health of a bank's loan portfolio, allowing for proactive risk management and strategic decision-making.
Different types of provisioning models
Various provisioning models are used in the banking industry, each with its own approach and methodology. The two primary types of provisioning models are the incurred loss model and the expected credit loss model.
The incurred loss model, also known as the historical loss model, has long been the traditional approach to provisioning in banking. Under this model, provisions are only made when a loss event has occurred. This model relies on historical data and past experiences to estimate future losses. While this model has been widely used, its main drawback is that it may not capture potential losses that have not yet materialised but are likely to occur in the future.
In contrast, the expected credit loss model takes a more forward-looking approach. It requires banks to estimate and provision for potential credit losses over the life of a loan, even if no loss event has occurred. This model considers a range of factors such as macroeconomic conditions, borrower-specific information, and expected changes in creditworthiness. By incorporating future expectations and forward-looking information, the expected credit loss model provides a more accurate assessment of potential credit losses.
Key factors to consider when choosing a provisioning model
When choosing a provisioning model, several key factors need to be considered to ensure its suitability for a banking institution. These factors include the regulatory environment, the loan portfolio's complexity, data availability, and the institution's risk appetite.
The regulatory environment plays a significant role in the selection of a provisioning model. Different jurisdictions have varying regulatory requirements and guidelines for provisioning. It is essential to understand and comply with these regulations to avoid penalties and ensure the institution's financial stability.
The complexity of the loan portfolio is another crucial factor to consider. A more sophisticated provisioning model, such as the expected credit loss model, may be more appropriate if a bank has a diverse portfolio with loans of varying types and risk profiles. On the other hand, if the loan portfolio is relatively simple, the incurred loss model may suffice.
Data availability and quality also play a vital role in provisioning model selection. Accurate and comprehensive data is essential for the successful implementation of provisioning models. Banks need to assess their data infrastructure and capabilities to determine whether they have the necessary data to support a particular model.
Lastly, the institution's risk appetite should be taken into account. Some provisioning models require a higher level of provisions to be set aside, which may impact the bank's profitability. Understanding the institution's risk appetite and aligning it with the chosen provisioning model is crucial for a successful implementation.
Pros and cons of different provisioning models
Each provisioning model has its advantages and disadvantages, and understanding these can help banks make informed decisions about which model to adopt.
The incurred loss model has the benefit of simplicity and ease of implementation. It relies on historical data, which is readily available, making it a practical choice for banks with limited data capabilities. However, the incurred loss model has its limitations. It may not adequately capture potential losses that have not yet materialised, leading to an underestimation of credit risk. This model also lacks the forward-looking nature of the expected credit loss model, which can be a disadvantage in an ever-changing economic environment.
On the other hand, the expected credit loss model offers several advantages. It takes a more proactive approach to risk management by considering future expectations and forward-looking information. By incorporating a range of factors, this model provides a more accurate estimation of potential credit losses. However, the expected credit loss model can be more complex to implement and may require more sophisticated data infrastructure and analytical capabilities. It also requires regular updates and adjustments to reflect changes in economic conditions and borrower-specific information.
Implementing a provisioning model in a banking institution
Implementing a provisioning model in a banking institution requires careful planning and coordination. The following steps can guide banks through the implementation process:
- Assess the current state: Before implementing a provisioning model, it is essential to assess the bank's current state, including its data infrastructure, risk management processes, and existing provisioning practices. This assessment will help identify any gaps or areas for improvement.
- Define the desired state: Once the current state has been assessed, banks should define the desired state, taking into account regulatory requirements, risk appetite, and the complexity of the loan portfolio. This step involves selecting the appropriate provisioning model and setting clear goals and objectives for implementation.
- Data preparation: Accurate and comprehensive data is crucial for successful provisioning model implementation. Banks need to ensure that their data is clean, consistent, and relevant. This may involve data cleansing, aggregation, and integration efforts to ensure the availability of high-quality data.
- Model development and validation: The next step is to develop the provisioning model based on the chosen approach. This involves building statistical models, defining key parameters and assumptions, and validating the model against historical data. Model validation is crucial to ensure the accuracy and reliability of the model's outputs.
- Testing and calibration: Once the model has been developed and validated, it should be calibrated using various scenarios and stress tests. This step helps assess the model's performance under different conditions and ensures its robustness.
- Implementation and monitoring: The provisioning model can be implemented after testing and calibration. Banks should establish clear processes and procedures for provisioning, including data collection, model execution, and reporting. Regular monitoring and review of the model's performance is essential to identify any issues or areas for improvement.
Best practices for successful provisioning model implementation
Implementing a provisioning model successfully requires adherence to best practices. Here are some key considerations to ensure a smooth implementation:
- Strong governance: Establishing a strong governance framework is crucial for effective provisioning model implementation. This includes clear roles and responsibilities, oversight from senior management, and regular reporting and communication.
- Clear documentation: Documenting all aspects of the provisioning model, including model development, validation, and implementation processes, is essential for transparency and auditability. Clear documentation ensures that internal and external stakeholders can easily understand and review the model.
- Robust data management: Accurate and reliable data is the foundation of any provisioning model. Banks should invest in robust data management systems and processes to ensure the availability and quality of data. This may involve data cleansing, integration, and validation efforts to mitigate any data-related risks.
- Regular model validation: Ongoing model validation is crucial to ensure the accuracy and reliability of the provisioning model. Banks should establish a regular validation process, including periodic reviews, sensitivity analyses, and benchmarking against industry standards.
- Training and awareness: Provisioning model implementation involves a significant change in processes and practices. Banks should provide comprehensive training and awareness programs to ensure staff understand the model's objectives, assumptions, and limitations. This will help facilitate a smooth transition and ensure effective utilisation of the model.
Challenges and potential pitfalls of provisioning models in banking
While provisioning models offer significant benefits, they also present challenges and potential pitfalls that banks should be aware of. Some common challenges include:
- Data quality and availability: Accurate and comprehensive data is crucial for successful provisioning model implementation. Banks may face challenges in sourcing the necessary data, ensuring its quality, and integrating it into the model.
- Model complexity: Some provisioning models, such as the expected credit loss model, can be complex and require sophisticated analytical capabilities. Banks may face challenges in building, validating, and maintaining these models, particularly if they lack the necessary expertise or resources.
- Regulatory compliance: Regulatory requirements for provisioning can be complex and subject to change. Banks need to stay updated with the latest regulations and ensure compliance, which may require significant efforts and resources.
- Model performance and stability: Provisioning models need to be regularly monitored and reviewed to ensure their performance and stability. Banks should establish robust monitoring processes and conduct regular model validations to identify any issues or areas for improvement.
Conclusion: Key takeaways and recommendations for navigating provisioning models in banking
Provisioning models play a crucial role in managing credit risk and ensuring financial stability in the banking industry. Understanding the different types of provisioning models, their pros and cons, and the key factors to consider when choosing a model is essential for successful implementation. By following best practices, banks can navigate the challenges and potential pitfalls associated with provisioning models and optimise their risk management strategies.
To navigate the world of provisioning models effectively, banking professionals and executives should:
- Evaluate the regulatory environment and align with regulatory requirements.
- Assess the complexity of their loan portfolio and choose a provisioning model that suits their needs.
- Ensure the availability and quality of data to support the chosen provisioning model.
- Consider the institution's risk appetite and align it with the selected model.
- Establish a robust implementation process, including model development, validation, and calibration.
- Adhere to best practices for successful provisioning model implementation, including strong governance, clear documentation, and robust data management.
- Regularly monitor and review the provisioning model's performance to identify any issues or areas for improvement.
By following these recommendations, banking institutions can confidently navigate the world of provisioning models, enhancing their risk management practices and ensuring financial stability in an ever-changing banking landscape.
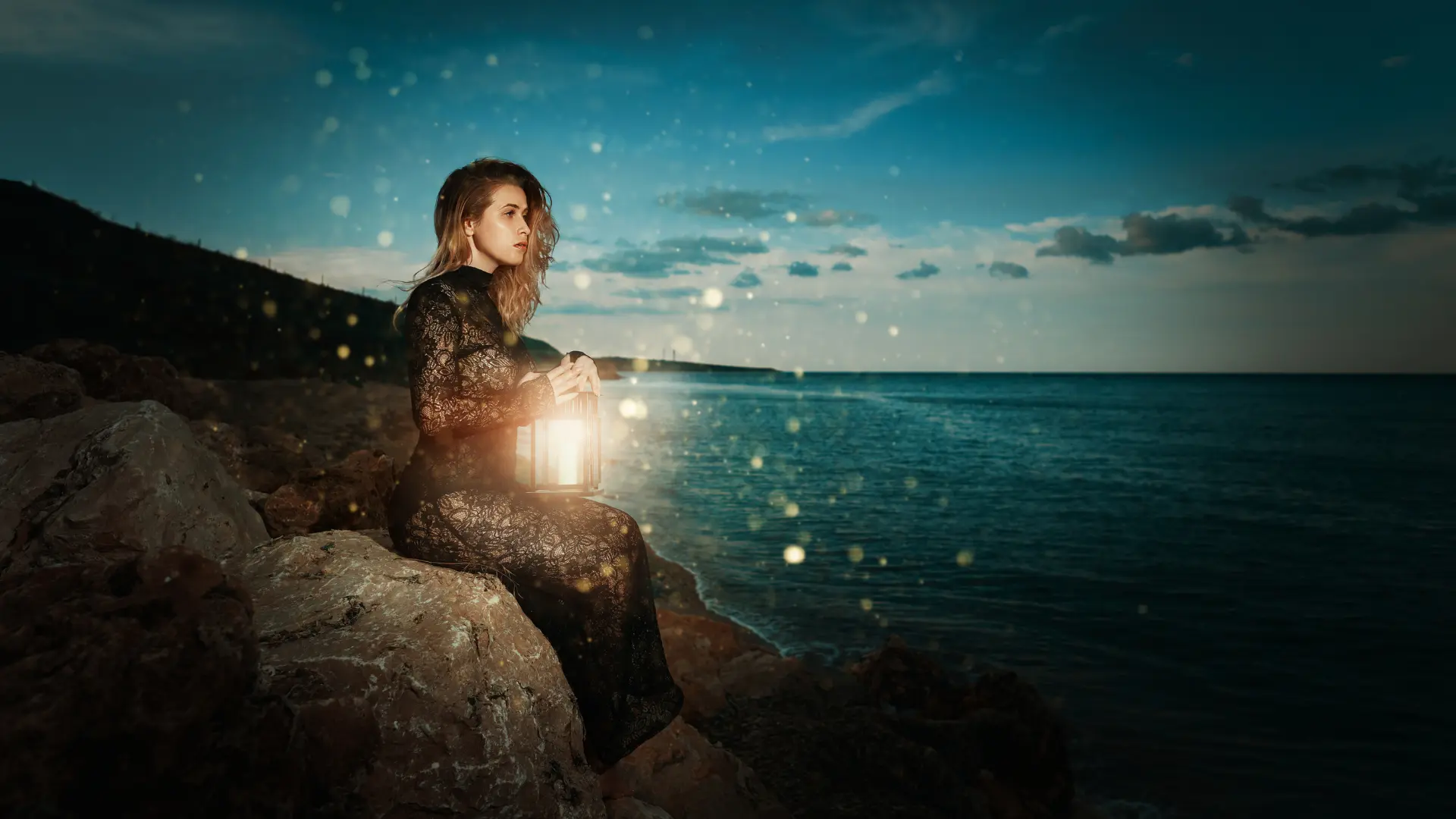